Getty Images
Since the dawn of the iPhone, many of the smarts in smartphones have come from elsewhere: the corporate computers known as the cloud. Mobile apps sent user data cloudward for useful tasks like transcribing speech or suggesting message replies. Now Apple and Google say smartphones are smart enough to do some crucial and sensitive machine-learning tasks like those on their own.
At Apple’s WWDC event this month, the company said its virtual assistant Siri will transcribe speech without tapping the cloud in some languages on recent and future iPhones and iPads. During its own I/O developer event last month, Google said the latest version of its Android operating system has a feature dedicated to secure, on-device processing of sensitive data, called the Private Compute Core. Its initial uses include powering the version of the company’s Smart Reply feature built into its mobile keyboard that can suggest responses to incoming messages.
Apple and Google both say on-device machine learning offers more privacy and snappier apps. Not transmitting personal data cuts the risk of exposure and saves time spent waiting for data to traverse the internet. At the same time, keeping data on devices aligns with the tech giants’ long-term interest in keeping consumers bound into their ecosystems. People that hear their data can be processed more privately might become more willing to agree to share more data.
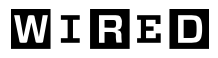
The companies’ recent promotion of on-device machine learning comes after years of work on technology to constrain the data their clouds can “see.”
In 2014, Google started gathering some data on Chrome browser usage through a technique called differential privacy, which adds noise to harvested data in ways that restrict what those samples reveal about individuals. Apple has used the technique on data gathered from phones to inform emoji and typing predictions and for web browsing data.
More recently, both companies have adopted a technology called federated learning. It allows a cloud-based machine-learning system to be updated without scooping in raw data; instead, individual devices process data locally and share only digested updates. As with differential privacy, the companies have discussed using federated learning only in limited cases. Google has used the technique to keep its mobile typing predictions up to date with language trends; Apple has published research on using it to update speech-recognition models.
Rachel Cummings, an assistant professor at Columbia who has previously consulted on privacy for Apple, says the rapid shift to do some machine learning on phones has been striking. “It’s incredibly rare to see something going from the first conception to being deployed at scale in so few years,” she says.
That progress has required not just advances in computer science but for companies to take on the practical challenges of processing data on devices owned by consumers. Google has said that its federated learning system only taps users’ devices when they are plugged in, idle, and on a free Internet connection. The technique was enabled in part by improvements in the power of mobile processors.
Beefier mobile hardware also contributed to Google’s 2019 announcement that voice recognition for its virtual assistant on Pixel devices would be wholly on-device, free from the crutch of the cloud. Apple’s new on-device voice recognition for Siri, announced at WWDC this month, will use the “neural engine” the company added to its mobile processors to power up machine-learning algorithms.
The technical feats are impressive. It’s debatable how much they will meaningfully change users’ relationship with tech giants.
Presenters at Apple’s WWDC said Siri’s new design was a “major update to privacy” that addressed the risk associated with accidentally transmitting audio to the cloud, saying that was users’ largest privacy concern about voice assistants. Some Siri commands—such as setting timers—can be recognized wholly locally, making for a speedy response. Yet in many cases transcribed commands to Siri—presumably including from accidental recordings—will be sent to Apple servers for software to decode and respond. Siri voice transcription will still be cloud-based for HomePod smart speakers commonly installed in bedrooms and kitchens, where accidental recording can be more concerning.
Google also promotes on-device data processing as a privacy win and has signaled it will expand the practice. The company expects partners such as Samsung that use its Android operating system to adopt the new Privacy Compute Core and use it for features that rely on sensitive data.
Google has also made local analysis of browsing data a feature of its proposal for reinventing online ad targeting, dubbed FLoC and claimed to be more private. Academics and some rival tech companies have said the design is likely to help Google consolidate its dominance of online ads by making targeting more difficult for other companies.
Michael Veale, a lecturer in digital rights at University College London, says on-device data processing can be a good thing but adds that the way tech companies promote it shows they are primarily motivated by a desire to keep people tied into lucrative digital ecosystems.
“Privacy gets confused with keeping data confidential, but it’s also about limiting power,” says Veale. “If you’re a big tech company and manage to reframe privacy as only confidentiality of data, that allows you to continue business as normal and gives you license to operate.”
A Google spokesperson said the company “builds for privacy everywhere computing happens” and that data sent to the Private Compute Core for processing “needs to be tied to user value.” Apple did not respond to a request for comment.
Cummings of Columbia says new privacy techniques and the way companies market them add complexity to the trade-offs of digital life. Over recent years, as machine learning has become more widely deployed, tech companies have steadily expanded the range of data they collect and analyze. There is evidence some consumers misunderstand the privacy protections trumpeted by tech giants.
A forthcoming survey study from Cummings and collaborators at Boston University and the Max Planck Institute showed descriptions of differential privacy drawn from tech companies, media, and academics to 675 Americans. Hearing about the technique made people about twice as likely to report they would be willing to share data. But there was evidence that descriptions of differential privacy’s benefits also encouraged unrealistic expectations. One-fifth of respondents expected their data to be protected against law enforcement searches, something differential privacy does not do. Apple’s and Google’s latest proclamations about on-device data processing may bring new opportunities for misunderstandings.
This story originally appeared on wired.com.